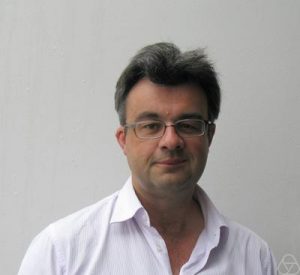
Emmanuel Candès (Source Wikimedia)
Emmanuel Candès has won a prestigious MacArthur Fellowship. The official announcement is here. The LA Times has a nice write-up. Both the Los Angeles Times and the MacArthur announcement highlight Candès’s work on compressed sensing. Terry Tao has a spot-on reaction to this work, quoted in the LA Times, typical of most mathematicians when you first hear about the method: you can’t be getting be getting something for nothing. This can’t work. But it does! Tao finally came around to believe it, as has the rest of the world.
Candès is a collaborative researcher. The work on compressed sensing came out of conversations he was having initially with researchers in imaging science (MRIs). He tested out his ideas in conversations with Tao (while picking up their children at daycare, according to the legend). In MathSciNet, you can see that Candès has 48 coauthors, representing a wide variety of people: statisticians, analysts, younger collaborators, older collaborators.
One of his key publications on compressed sensing is his paper with Justin Romberg and Terry Tao in Communications on Pure and Applied Mathematics, Stable signal recovery from incomplete and inaccurate measurements. For your reading enjoyment, our review of it is reproduced below. The complete article is here.
Congratulations, Emmanuel Candès!
MR2230846
Candès, Emmanuel J.(1-CAIT-ACM); Romberg, Justin K.(1-CAIT-ACM); Tao, Terence(1-UCLA)
Stable signal recovery from incomplete and inaccurate measurements.
Comm. Pure Appl. Math. 59 (2006), no. 8, 1207–1223.
94A12
The authors consider the problem of recovering an unknown sparse signal $x_0(t)inBbb{R}^m$ from $nll m$ linear measurements which are corrupted by noise, building on related results in [E. J. Candès and J. K. Romberg, Found. Comput. Math. 6 (2006), no. 2, 227–254; MR2228740; E. J. Candès, J. K. Romberg and T. C. Tao, IEEE Trans. Inform. Theory 52 (2006), no. 2, 489–509; MR2236170; E. J. Candès and T. C. Tao, IEEE Trans. Inform. Theory 51 (2005), no. 12, 4203–4215; MR2243152; “Near optimal signal recovery from random projections: universal encoding strategies?”, preprint, arxiv.org/abs/math.CA/0410542, IEEE Trans. Inform. Theory, submitted; D. L. Donoho, Comm. Pure Appl. Math. 59 (2006), no. 6, 797–829; MR2217606]. Here $x_0(t)$is said to be sparse if its support $T_{0}=lbrace tcolon x_0(t)neq0rbrace$ has small cardinality. The measurements are assumed to be of the form $y=Ax_0+ e$, where $A$ is the $ntimes m$ measurement matrix and the error term $e$ satisfies $Vert eVert_{l_{2}}leepsilon$. Given this setup, consider the convex program $$ minVert xVert_{l_{1}} {rm subject to};Vert Ax-y Vert_{l_{2}}leepsilon.;;;(textrm {P}_{2}) $$
The authors define $A_{T}$, $Tsubsetlbrace 1,ldots,mrbrace$, to be the $ntimesvert Tvert$ submatrix obtained by extracting the columns of $A$ corresponding to the indices in $T$. Their results are stated in terms of the $S$-restricted isometry constant $delta_S$ of $A$ [E. J. Candès and T. C. Tao, op. cit., 2005], which is the smallest quantity such that $$ (1-delta_{S})Vert cVert_{l_{2}}^{2}leVert A_{T}c Vert_{l_{2}}^{2}le(1+delta_{S})Vert cVert_{l_{2}}^2 $$ for all subsets $T$ with $vert Tvertle S$ and coefficient sequences $(c_{j})_{jin T}$.
Their main results are below. The first describes stable recovery of sparse signals, while the second yields stable recovery for approximately sparse signals by focusing on the $S$ largest components of the signal $x_0$.
Theorem 1. Let $S$ be such that $delta_{3S}+3delta_{4S}<2$. Then for any signal $x_{0}$ supported on $T_0$ with $vert T_0vertle S$ and any perturbation $e$ with $Vert eVert_{l_{2}}leepsilon$, the solution $x^{sharp}$ to $({rm P}_2)$ obeys $$ vert x^{sharp}-x_0vert_{l_{2}}le C_{S}cdotepsilon, $$ where the constant $C_S$ depends only on $delta_{4S}$. For reasonable values of $delta_{4S}$, $C_S$ is well behaved; for example, $C_Sapprox8.82$ for $delta_{4S}=frac{1}{5}$ and $C_{S}approx 10.47$ for $delta_{4S}=frac{1}{4}$.
Theorem 2. Suppose that $x_0$ is an arbitrary vector in $Bbb{R}^m$, and let $x_{0,S}$ be the truncated vector corresponding to the $S$ largest values of $x_0$ (in absolute value). Under the hypotheses of Theorem 1 in the paper, the solution $x^{sharp}$ to $({rm P}_{2})$ obeys $$ Vert x^{sharp}-x_0Vert_{l_{2}}le C_{1,S}cdotepsilon+ C_{2,S}cdotfrac{Vert x_0-x_{0,S}Vert_{l_{1}}}{sqrt{S}}. $$
For reasonable values of $delta_{4S}$, the constants in the equation labelled 1.4 in the paper are well behaved; for example, $C_{1,S}approx 12.04$ and $C_{2,S}approx8.77$ for $delta_{4S}=frac{1}{5}$.
Reviewed by Brody Dylan Johnson
This type of great research works because of the adherence to standards and excellence of each of the contributors and constructs like commutative properties in linear algebraic functions. Also advances in infrastructure, technology, and sound foundational architecture development of frameworks are an enabler and constant for any research done these days. Cheers to the author and congratulations.